Iterative Orientation Tuning for Contrast Detection in Images
by Marina Kolesnik
A biologically-motivated model for contrast detection in images - the iterative orientation tuning model - has been developed at the Fraunhofer Institute for Media Communication. The model has been inspired by a regular orientation preference layout of simple cells in the primary visual cortex of mammals. The model allows the efficient detection of contours in complex images.
About forty years ago, Hubel and Wiesel discovered that simple cells in cat primary visual cortex (V1) are tuned for the orientation of light/dark borders. The inputs to V1 come from the lateral geniculate nucleus (LGN), whose cells are not significantly orientation selective. The orientation selectivity of simple cells in V1, as proposed by Hubel and Wiesel, is derived from an oriented arrangement of the input from the LGN. Namely, ON-centre LGN inputs have receptive fields (RFs) centres aligned over simple cell's ON subregions, and similarly for OFF-centre inputs. Because of this input arrangement, simple cells perform a linear spatial summation of light intensity in their fields, and their RFs have an elongated shape. The orientation preference of simple cells is quite narrow, and turning a bar-stimulus through more than 20 degrees from the preferred orientation greatly reduces the cell's firing rate.
Although many aspects of simple cell responses are consistent with this linear model, there also are important violations of linearity. For example, scaling the contrast of a stimulus would identically scale the responses of a linear cell. At high contrasts, however, the responses of simple cells show clear saturation. Such behaviour of the simple cells is referred to as contrast invariance of orientation selectivity.
Several computational models have applied the principle of orientation selectivity of simple cells for the processing of natural images generating a pattern of image contours. In fact, any model emulating the functionality of simple cells, has the property of an efficient edge detector. But what is the role of the impressive regularity of simple cells in V1 for the processing of visual stimuli?
Simple cells in V1 are aligned into ocular dominance stripes. When the orientation preference of cells in the ocular dominance stripes was related to their position, an astonishingly systematic organisation emerged: the orientation preference changed linearly with its position across V1. Because, furthermore, the orientation columns tended to be at right angles to ocular dominance stripes, their regular layout was nicknamed the Icecube layout.
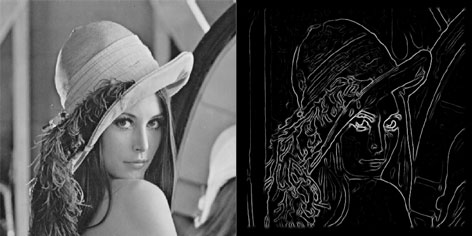 |
Figure 1: Natural input image and the edge image with eyes enhanced via attentional feedback projection to the LGN. |
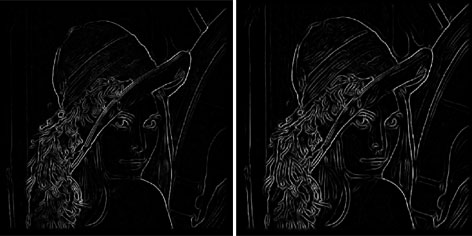 |
Figure 2: Edge images at 1st and 5th iteration. Closer visual inspection reveals how some initially weak edges become visible at 5th iteration while others, situated in the vicinity of strong edges and visible at 1st iteration, become suppressed and vanish. |
We have suggested a model for iterative orientation tuning, which explicitly involves the morphology of simple cells for the processing of edges in images. In this model, an activated simple cell amplifies the activity of proximate cells, so that these are becoming locally tuned for distinguished orientation. After several cycles of iterative tuning, the response of simple cells to visual input stabilises. The model architecture is built upon a simple cell circuit composed of segregated ON- and OFF-data streams interacting via a mechanism of opponent inhibition. There are two types of local intracortical interaction each is governed by the spatial layout of simple cells: 1) amplification of the activity level of simple cells that are spatially close within the Icecube layout, and 2) cross-orientation inhibition. The latter is based on considerable experimental evidence suggesting that stimuli at non-optimal orientations suppress the background activity of simple cells.
The iterative amplification of nearby cells enhances responses of both retinotopically proximate cells and cells tuned for similar orientations activating a process of selective orientation tuning. The cross-orientation inhibition affects the activity of simple cells in two ways. On the one hand, the activity is cancelled out by strongly activated retinotopically close cells of orthogonal orientation. On the other hand, the response of strongly activated cells will only be slightly inhibited by retinotopically close cells of orthogonal orientation if these are slightly activated. It follows that the cross-oriented inhibition has the potential to suppress weak responses in the vicinity of contrastive edges and increase model's robustness to noise.
Testing the iterative orientation tuning model with different images, revealed several remarkable properties:
1.The model's response to grey scale images is a fine pattern of image contours. Because of the favourable amplification of weak contrast variations over the strong ones at subsequent iterations, the iterative model is responsible for about 70% of contrast invariance of orientation selectivity.
2.The iterative amplification enhances the response to weak isolated contrast variations. The same level of contrast variations in the vicinity of strong edges does not trigger the amplification. In a sense, the model's response becomes locally attuned to distinguished orientations in the visual pattern. This capability of adjusting the response to either strong or weak contrast changes seems to be natural for biological systems (see Figure 2).
3.The model is highly robust to noise. Noise reduction is especially pronounced in the vicinity of sharp edges, where weak responses are 'cancelled out' by stronger responses due to local interaction.
4.All computational stages employ the local processing. This would make transition to parallel computations an easy task.
5. The computational scheme is flexible and can be extended by incorporating the response of other orientation selective cells, such as complex cells, or color-opponent cells. Further incorporation of memory association feedback mediated by projections to the LGN would turn the detection of contours into a selective process enhancing the response to edges of interest (see Figure 1).
The model's performance gives a powerful demonstration of how the mechanisms of neuronal visual processing, and the morphology of visual neurons can be exploited for the development of advanced image processing algorithms.
Link:
http://viswiz.imk.fraunhofer.de/~marina/
Please contact:
Marina Kolesnik, Fraunhofer IMK
Tel: +49 2241 14-3421
E-mail: marina.kolesnik@imk.fraunhofer.de
|