Searching and Browsing Databases of Digital Decoration Designs
by Mark Huiskes and Eric Pauwels
The FOUNDIT project, coordinated by CWI, aims to develop content-based image retrieval systems particularly geared towards requirements for browsing and searching digital decoration designs. Research is currently directed towards the construction of automatic design descriptions in MPEG-7. Results of this project are currently being tested in collaboration with an Italian fashion designer.
Content-based image retrieval (CBIR) through the searching or browsing of databases of decorative designs such as clothes, textiles and wallpaper remains a challenging problem, especially when the user's subjective appreciation is involved. In these applications, the only way to elucidate the user's preference is by continuously soliciting his/her feedback. This feedback is then harnessed to estimate for each image in the database the likelihood of its relevance with respect to the user's goals whereupon the most promising candidates are displayed for further inspection and feedback.
The goal of the FOUNDIT project is to build a CBIR search engine based on sound principles of inference that can handle the requirements typically encountered in decoration-related image and design databases. The FOUNDIT system comprises the following three modules. The graphically oriented interface (see Figure 1) allows the user to provide the system with relevance feedback by selecting examples and counter-examples which are collected in separate bins. By coupling it to mathematical features, this qualitative feedback is then transformed by the relevance inference engine into a probabilistic measure for each image in the database. The inference engine therefore relies on the availability of pre-computed features that characterise the visual appearance of the images. This feature database is generated off-line by the feature extraction engine.
The feature extraction engine consists of a large collection of algorithms for quantitative image characterisation. The routines are not restricted to computation of low-level features such as global colour and texture measures, but try to establish a link to the more semantically meaningful categories that are typically used by humans when making aesthetical judgments on designs.
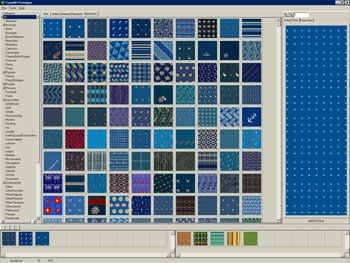 |
Figure 1: Screenshot of the FOUNDIT prototype interface. Feedback is supplied by selecting positive and negative examples which are collected on the display bar at the bottom (positive examples on the left, negative on the right). |
In recognition of their vital role in capturing the essence of a design, much effort has been directed towards the detection of so-called salient design elements. Two main strategies are followed to this end: figure-ground segregation (see Figure 2), and grouping of primitives. The figure-ground segregation is based on colour-texture region extraction and subsequent region classification based on regional property variables such as relative size, connectedness and compactness. Primitive grouping is directed at finding objects by analysing the configurations of primitive image elements such as edges. In this manner we may, for instance, detect the occurrence and arrangement of homogeneous strips. Based on the decomposition of a design into a ground and one or more salient regions or objects, the feature computation process can be further specialised. For salient objects we compute, among other properties, size, orientation, colour and shape (region and contour-based), and in case of several objects, spatial organisation, occurrence of periodic patterns and motif variation (colour, shape, orientation). The background regions may be similarly characterised, for instance, in terms of colour and textural properties.
Based on the various elements, properties and relationships thus obtained, it becomes feasible to construct an automatic interpretation of the design. The MPEG-7 semantic content-description schemes provide a convenient framework to this end.
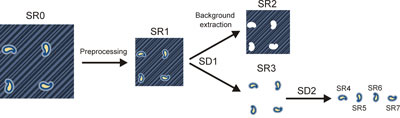 |
Figure 2: Example of a design segment decomposition using the MPEG-7 StillRegion (SR) and SegmentDecomposition (SD) Description Schemes. The boxes represent semantical entities, the arrows semantical relations. |
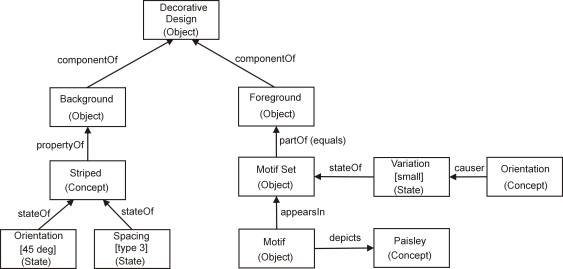 |
Figure 3: Example of a semantic content description of the design shown in Figure 2. |
An example of a semantic content description is shown in Figure 3 for the decorative design of Figure 2. The decorative design consists of a background and a foreground entity. The background is associated with the abstract concept of 'striped-ness'. Two state entities further specify the quality of the stripes (their orientation and their size). The foreground consists of a set of motifs. The type of motif set may be further clarified by a variation state (indicating to what extent the motifs vary within the set). The motifs that occur within the set, in this case so-called paisley motifs, may then be described independently.
The FOUNDIT Project is partially supported by the European Commission under the IST Programme of the Fifth Framework.
Link:
http://www.cwi.nl/~foundit
Please contact:
Eric Pauwels, CWI
Tel: +31 20 592 4225
E-mail: Eric.Pauwels@cwi.nl
|